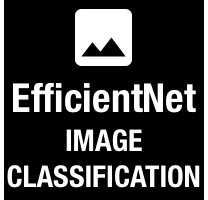
EfficientNet
EfficientNet is a powerful neural network for image classification tasks.
π·οΈ Price not available
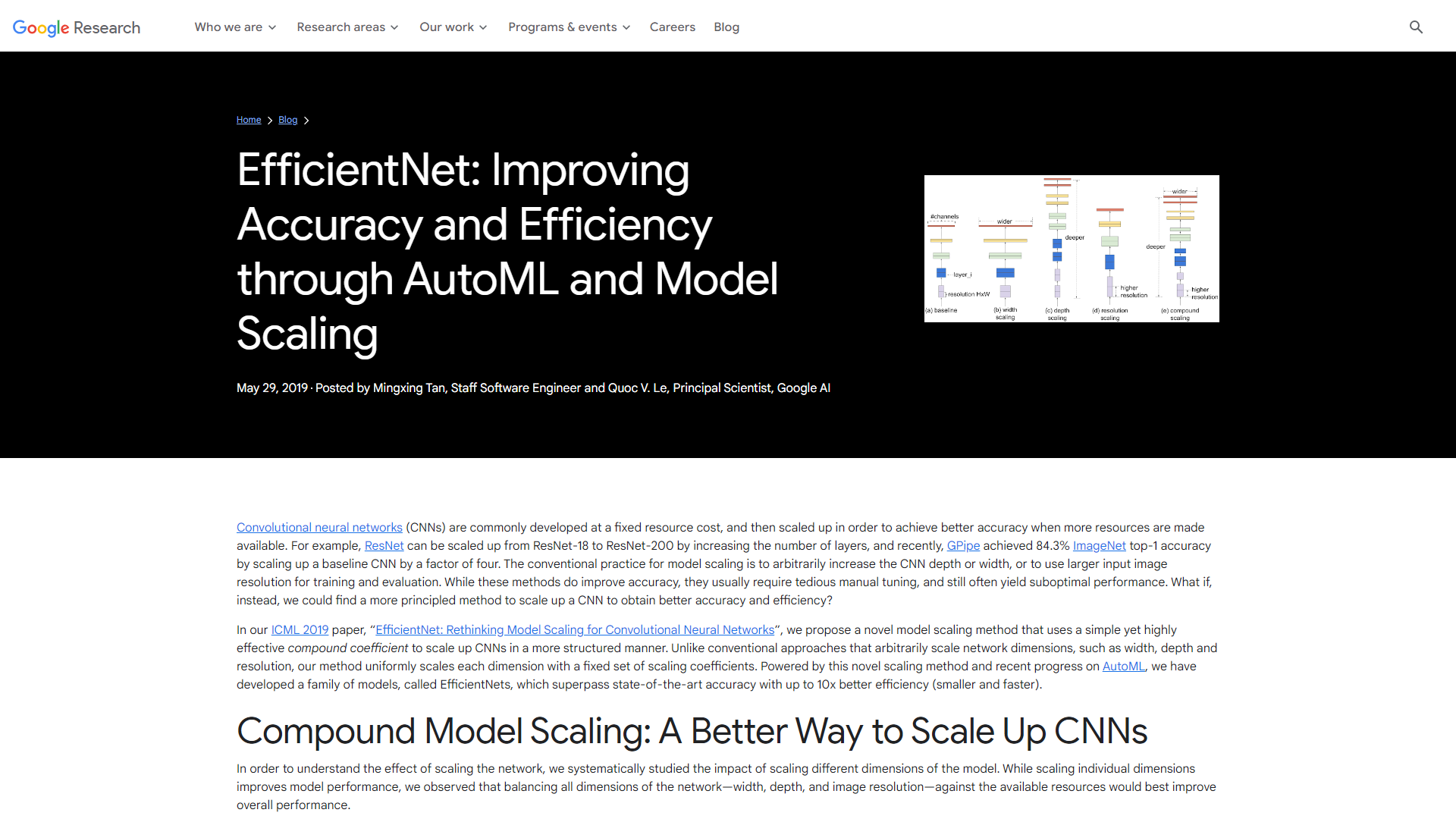
- Overview
- Pricing
- Features
- Pros
- Cons
Overviewβ
EfficientNet is an advanced deep learning model designed for image recognition. Developed by Google AI, it uses a new scaling method that balances depth, width, and resolution to improve accuracy. This model has become popular because it achieves high performance with fewer parameters compared to traditional models, making it efficient in both training and inference.
The architecture of EfficientNet is based on a family of models, where each model in the family improves upon the previous one. This scaling method allows users to choose the level of performance they need, from lighter models that are faster to heavier ones that achieve higher accuracy. EfficientNet is versatile and can be used in various applications, from mobile devices to large servers.
Overall, EfficientNet represents a significant step forward in the field of computer vision, combining efficiency and high accuracy. Whether you are a researcher or a developer, this model provides an effective solution for tasks requiring image classification, enabling the use of deep learning in a wider range of applications.
Pricingβ
Plan | Price | Description |
---|
Key Featuresβ
π― Model Scaling: EfficientNet scales models in a balanced way by adjusting depth, width, and resolution.
π― High Accuracy: Delivers state-of-the-art accuracy on common image classification tasks.
π― Fewer Parameters: Requires significantly fewer parameters than other models, making it easier to train.
π― Multi-Task Learning: Can be fine-tuned for other tasks beyond image classification.
π― Versatile Applications: Suitable for both mobile and cloud-based applications.
π― Pre-trained Models: Available pre-trained models for quick implementation and experimentation.
π― Support for Transfer Learning: Facilitates transfer learning to boost performance on specific tasks.
π― Robust Performance: Maintains strong performance across various datasets and benchmarks.
Prosβ
βοΈ Efficient Use of Resources: Saves computational power and memory, allowing for broader accessibility.
βοΈ Flexible: Can be scaled according to project requirements, fitting different levels of performance needs.
βοΈ High Performance: Achieves excellent results on several benchmarks in image classification tasks.
βοΈ User-Friendly: Encourages developers with easy access to pre-trained models for quicker deployment.
βοΈ Strong Community Support: Backed by Google and a large community, facilitating updates and improvements.
Consβ
β Complexity: The scaling method may be difficult for beginners to understand and implement.
β Dependency on Data: Performance is highly dependent on the quality and size of the training dataset.
β Overfitting Risk: Some larger models might overfit on smaller datasets if not properly managed.
β Resource Intensive: While efficient, some of the larger models require significant resources for training.
β Limited Out-of-the-Box Performance: May need fine-tuning to reach optimal performance on specific tasks.
Manage projects with Workfeed
Workfeed is the project management platform that helps small teams move faster and make more progress than they ever thought possible.
Get Started - It's FREE* No credit card required
Frequently Asked Questionsβ
Here are some frequently asked questions about EfficientNet. If you have any other questions, feel free to contact us.