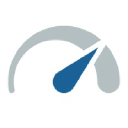
MLPerf
MLPerf is a benchmark suite for machine learning performance.
π·οΈ Price not available
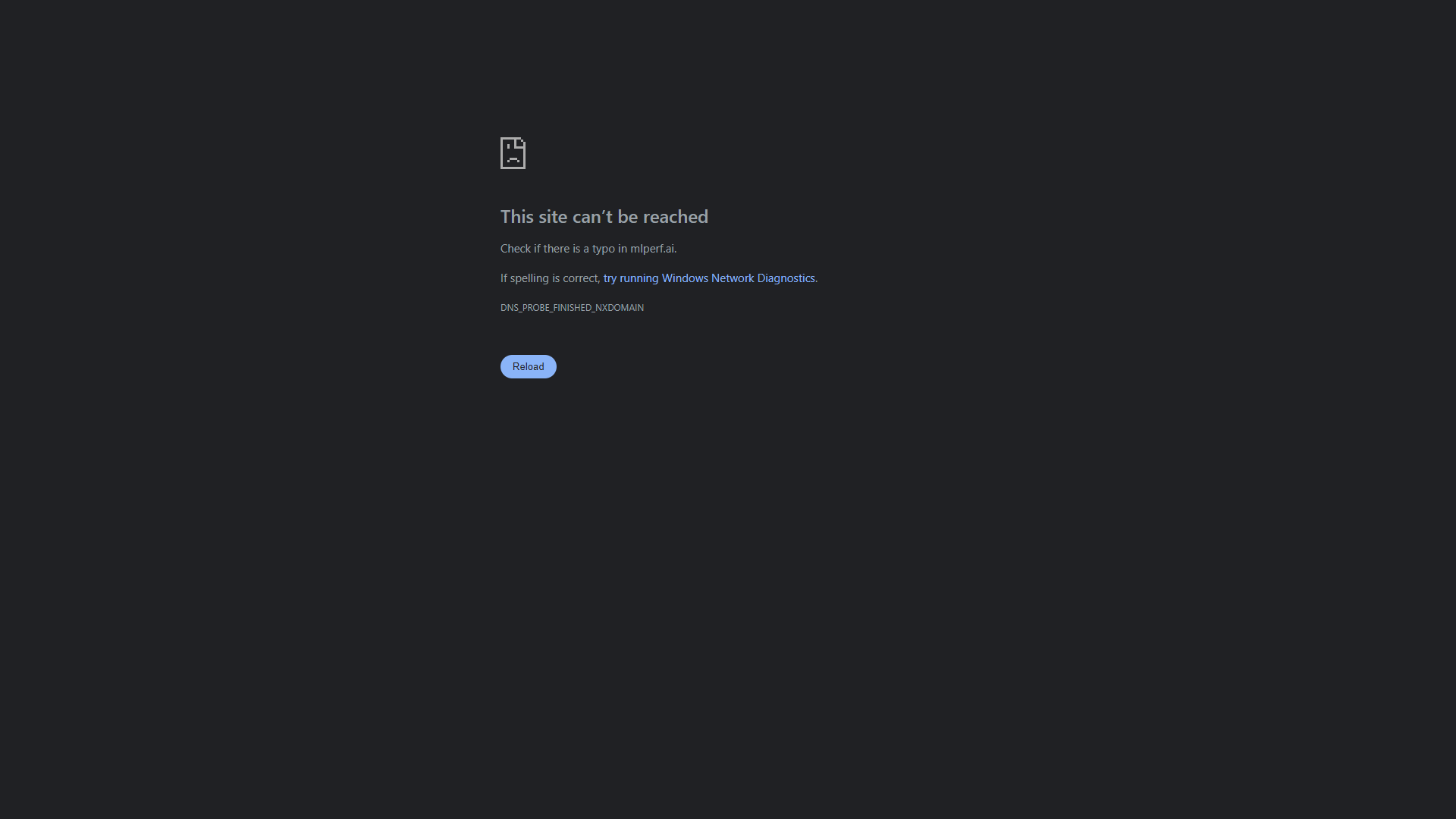
- Overview
- Pricing
- Features
- Pros
- Cons
Overviewβ
MLPerf is a benchmark that evaluates and measures the performance of machine learning hardware, software, and services. Developed by a group of industry experts, it aims to provide a fair and consistent way to compare various machine learning systems. The benchmarks cover different aspects of machine learning, ensuring that a wide range of tasks are considered in the evaluation process. This suite of tests is used by companies and researchers to gain insights into how well their machine learning solutions perform. By using MLPerf, organizations can make informed decisions about what hardware and software are best suited for their specific needs. This can lead to better performance, efficiency, and cost-effectiveness in deploying machine learning applications. Additionally, MLPerf fosters competition in the industry, encouraging vendors to improve their products based on benchmarking results. This drive for improvement can ultimately lead to faster advancements in machine learning technology, benefitting everyone from researchers to businesses deploying AI solutions.
Pricingβ
Plan | Price | Description |
---|
Key Featuresβ
π― Comprehensive Benchmarks: MLPerf features a variety of benchmarks that assess different aspects of machine learning tasks.
π― Open Source: The benchmarks are available for everyone to use, promoting transparency and collaboration in the ML community.
π― Regular Updates: MLPerf continuously updates its benchmarks to reflect the latest trends and technologies in machine learning.
π― Wide Adoption: Many leading tech companies use MLPerf, making it a trusted standard in evaluating machine learning performance.
π― Support for Multiple Frameworks: The suite supports popular machine learning frameworks like TensorFlow, PyTorch, and MXNet.
π― Detailed Reporting: MLPerf provides detailed reports of benchmark results, helping users understand performance metrics.
π― Scalable: The benchmarks can be run on various systems, from edge devices to large cloud-based environments.
π― Community Engagement: MLPerf encourages community contributions, fostering collective improvement in benchmarking methods.
Prosβ
βοΈ Industry Standard: Being widely adopted, MLPerf results are recognized across the machine learning community.
βοΈ Fair Comparison: The standardized benchmarks allow for fair comparisons between different solutions.
βοΈ Encourages Innovation: The competitive nature of the benchmarks drives companies to innovate and improve their offerings.
βοΈ Resource Availability: The open-source nature ensures resources are available for all users.
βοΈ Insightful Metrics: Comprehensive reports provide valuable insights into performance, helping optimize ML deployments.
Consβ
β Complex Setup: Setting up MLPerf can be complex for users without technical expertise.
β Limited Benchmarks: Some users may find that certain specialized tasks aren't covered in the benchmarks.
β Resource Intensive: Running the benchmarks can require significant computing resources.
β Potential for Misinterpretation: Results can sometimes be misinterpreted, leading to incorrect conclusions about performance.
β Steep Learning Curve: New users may face a learning curve in understanding the benchmarking framework.
Manage projects with Workfeed
Workfeed is the project management platform that helps small teams move faster and make more progress than they ever thought possible.
Get Started - It's FREE* No credit card required
Frequently Asked Questionsβ
Here are some frequently asked questions about MLPerf. If you have any other questions, feel free to contact us.