Project Forecasting with Data-Driven Insights
In today's fast-paced business environment, successful project management requires more than just experience and intuition. Data-driven insights are essential for accurate project forecasting, helping teams predict potential outcomes and make informed decisions. This article aims to illuminate effective strategies for utilizing data in forecasting, providing a blend of theory and practical examples to ensure a comprehensive understanding.
With the constant evolution of technology, organizations can harness vast amounts of data to enhance their project forecasting capabilities. The integration of data analytics, predictive modeling, and performance metrics can significantly improve project accuracy, reduce risks, and optimize resource allocation. Let's dive into key concepts that underpin effective project forecasting with data-driven insights.
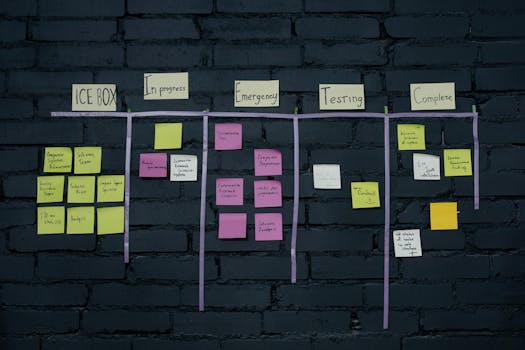
π Key Conceptsβ
1. Understanding Project Forecastingβ
Project forecasting involves predicting the future outcomes of a project, including timelines, budgets, and resource needs. Data-driven forecasting leverages historical data and analytics to enhance accuracy and reliability. Key methods include:
-
Quantitative forecasting: Uses numerical data and statistical techniques to predict outcomes. Techniques might include time series analysis and regression models.
-
Qualitative forecasting: Involves expert opinions and subjective assessments. While less data-oriented, it can provide valuable context and insights not captured by numbers.
2. The Role of Data in Forecastingβ
Data serves as the foundation for developing forecasting models. Consider the following dimensions of data:
-
Historical data: Past project performance metrics (cost, time, resources) can highlight trends and patterns. Analyzing historical results helps inform future predictions. ποΈ
-
Real-time data: Ongoing project data provides updates, allowing for adjustments in forecasting as the project progresses.
-
External data: Information about market trends, competitor performance, and economic conditions can influence project outcomes.
3. Predictive Analyticsβ
Predictive analytics utilizes statistical algorithms and machine learning techniques to identify trends and predict future events. Common tools include:
-
Regression analysis: Helps in understanding relationships between variables, such as budget and timelines. π
-
Time series forecasting: Analyzes data points collected over time to identify trends and seasonality.
-
Classification algorithms: Used to categorize data into defined classes, identifying risk factors that could impact project outcomes.
4. Key Performance Indicators (KPIs)β
KPIs are vital metrics that assess project performance and can be pivotal in forecasting. Some important KPIs include:
-
Schedule performance index (SPI): Compares the earned value against the planned value to assess time efficiency.
-
Cost performance index (CPI): Evaluates the project's cost efficiency by comparing earned value with actual costs.
-
Resource utilization: Measures how effectively resources are managed, influencing both cost and time forecasts.
π― How to Applyβ
Practical Steps for Effective Project Forecastingβ
-
π Step 1: Gather Historical Data
Collect historical data from previous projects similar in scope and size. Focus on key metrics like timelines, budgets, and resource usage to identify trends. -
π Step 2: Analyze Data
Apply statistical techniques to analyze historical data. Use software tools like Excel, R, or Python libraries (e.g., Pandas, NumPy) for regression analysis or time series forecasting. -
π‘ Step 3: Choose Appropriate Metrics
Decide which KPIs will help measure project performance. Ensure these align with overall project goals. -
π Step 4: Develop Predictive Models
Create models using algorithms appropriate to the data type. For instance, if you're working with historical cost data, regression analysis may be beneficial. If you're focused on timelines, consider time series methods. -
π Step 5: Conduct Scenarios Analysis
Perform scenario modeling to assess potential risks and uncertainties. This will help in understanding how different factors might impact project outcomes. -
π Step 6: Monitor Ongoing Performance
Throughout the project, continuously monitor performance against the established KPIs. Use real-time data to adjust forecasts and strategies accordingly. -
π Step 7: Review and Learn
After project completion, review forecasting accuracy. Analyze discrepancies between predictions and actual outcomes to improve future forecasting models.
Case Study: Implementing Data-Driven Forecasting in Practiceβ
Let's consider a mid-sized software development company, TechSoft, that implemented data-driven forecasting to enhance project accuracy.
-
Historical Data Gathering: TechSoft reviewed data from the last 20 software projects, focusing on timelines and budget overruns. They found an average delay of 20% across projects due to inconsistent resource allocation.
-
Data Analysis: The team used regression analysis to identify patterns in past delays associated with project complexity and resource allocation. This helped them to quantify risk factors.
-
KPI Establishment: They adopted SPI and CPI as primary KPIs to measure project performance effectively.
-
Predictive Modeling: TechSoft employed machine learning algorithms to develop predictive models. These models successfully identified potential bottlenecks and resource needs before project initiation.
-
Scenario Analysis: By simulating different project scenarios, TechSoft proactively identified risks, such as fluctuating client requirements and staffing issues.
-
Ongoing Monitoring: Throughout the project, the team utilized dashboards to visualize ongoing performance. This real-time data allowed them to adjust their strategies swiftly when deviations occurred.
-
Review and Learn: Post-project, TechSoft analyzed their forecasting results and discovered a 15% improvement in project delivery time due to data-driven insights. Lessons learned were documented and shared across teams for continuous improvement.
Manage projects with Workfeed
Workfeed is the project management platform that helps small teams move faster and make more progress than they ever thought possible.
Get Started - It's FREE* No credit card required
π οΈ Frequently Asked Questionsβ
Here are some frequently asked questions about "Project Forecasting with Data-Driven Insights".
π Conclusionβ
Project forecasting is an essential aspect of successful project management, and incorporating data-driven insights is key to enhancing accuracy and reliability. By systematically gathering and analyzing data, applying predictive analytics, and continually monitoring performance, organizations can significantly improve their forecasting capabilities. The case study of TechSoft serves as an excellent example of how the application of these theories can yield substantial benefits in real-life scenarios.
Adopting these strategies not only increases project success rates but also helps teams to become more agile in navigating uncertainties, ultimately driving efficiency and innovation. Embrace the power of data-driven insights in your project forecasting efforts and watch your projects flourish! π