Effective Strategies for Predictive Analytics in Project Scheduling
In todayβs fast-paced project environment, successful scheduling is crucial for delivering projects on time and within budget. With the ever-increasing complexity of project management, leveraging predictive analytics is a game changer. Predictive analytics uses historical data and statistical algorithms to forecast future outcomes. By integrating this powerful tool into project scheduling, managers can enhance decision-making, reduce risks, and optimize resource allocation.
This article delves into effective strategies for implementing predictive analytics in project scheduling. We will explore key concepts that underpin predictive analytics, provide practical examples, and outline actionable steps to maximize the benefits of this innovative approach. Are you ready to take your project scheduling to the next level? Let's dive in! π
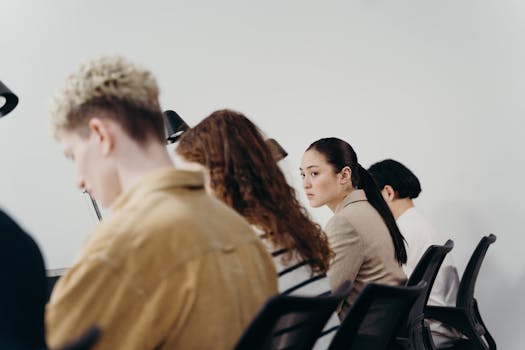
π Key Conceptsβ
What is Predictive Analytics?β
Predictive analytics is the practice of analyzing current and historical data to make informed predictions about future events. In the context of project scheduling, it involves understanding project patterns, identifying possible risks, and leveraging data trends to make proactive decisions. This helps in optimizing timelines and resource management.
Importance of Predictive Analytics in Project Schedulingβ
Predictive analytics plays a vital role in project scheduling by:
-
Risk Management: Identifying potential risks before they become critical issues. Analyzing historical data helps project managers pinpoint recurring problems and develop strategies to mitigate them.
-
Resource Optimization: Ensuring that resources are allocated efficiently. By forecasting the resource needs based on project timelines and historical usage patterns, projects can avoid overstaffing or underutilizing resources.
-
Enhanced Decision-Making: Facilitating better project planning strategies. Predictive analytics provides valuable insights that guide project managers in making data-driven decisions rather than relying on intuition alone.
-
Improving Project Outcomes: Enhancing the likelihood of meeting deadlines and staying within budget by allowing for proactive adjustments and course corrections.
Key Techniques in Predictive Analyticsβ
-
Regression Analysis: This technique helps identify relationships among variables. For instance, it can predict project duration based on different contributing factors like team size, deadlines, and budget constraints.
-
Time Series Analysis: By analyzing data points collected over time, time series analysis forecasts future values. This can help in understanding how long similar projects have taken in the past and predict timelines for future projects.
-
Machine Learning: Utilizing algorithms to identify patterns in large datasets, machine learning can improve the accuracy of predictions. It can analyze numerous factors affecting project success and suggest optimal scheduling strategies.
-
Simulation Models: These models can simulate different scheduling scenarios, allowing project managers to visualize the impact of various decisions before they are made.
π― How to Applyβ
To effectively implement predictive analytics in project scheduling, follow these practical steps:
π Step 1: Data Collectionβ
π Description: Collect relevant historical data related to past projects, such as timelines, budgets, resource usage, and any challenges faced.
Example:β
When managing a software development project, gather data from previous projects, including:
- Project duration
- Team size and composition
- Budget forecasts vs. actual spending
- Notable delays and their causes
π Step 2: Data Analysisβ
π οΈ Description: Use statistical techniques to analyze the collected data and identify patterns.
Example:β
Conduct a regression analysis to understand how factors like team size influence project completion time. For instance, you might discover that projects with larger teams often complete tasks faster, but at a certain point, more team members do not significantly improve efficiency.
π Step 3: Model Developmentβ
ποΈ Description: Develop predictive models that can illustrate how various factors will impact future projects.
Example:β
Using machine learning, create a model that predicts project costs based on historical data. You may find that additional features, like the complexity of tasks, significantly affect final costs.
π Step 4: Scenario Simulationβ
π Description: Run simulations based on the developed models to test various scenarios.
Example:β
Simulate different viewpoints such as increasing the team size or altering deadlines. This can help estimate how changes affect project timelines and budgets. Tools like Monte Carlo simulations can be particularly useful here!
π Step 5: Decision Makingβ
π― Description: Utilize insights and predictions to make informed scheduling decisions.
Example:β
If your simulation indicates that adding one more developer on a tight project timeline could prevent a delay, consider adjusting roles to optimize project completion efficiently.
π Step 6: Continuous Monitoring and Refinementβ
π Description: Regularly monitor project progress and refine your predictive models with fresh data.
Example:β
In the current project, frequently assess how actual timelines compare to your forecasts. Update your predictive models accordingly for more accurate future estimations.
π Tools for Predictive Analyticsβ
- Microsoft Project: It offers scheduling tools and can integrate with other data analytic solutions.
- Primavera P6: Known for its project management capabilities, this tool also supports predictive analytics.
- Tableau: For data visualization and predictive reporting, Tableau provides insights that can greatly inform project scheduling.
π Conclusionβ
Leveraging predictive analytics in project scheduling is no longer a luxury; itβs a necessity for project managers aiming for success in todayβs competitive landscape. By understanding key concepts, implementing actionable strategies, and utilizing a variety of tools, you can enhance your project management processes significantly.
Donβt delay! Start incorporating predictive analytics into your workflow and watch your projects thrive! π
Manage projects with Workfeed
Workfeed is the project management platform that helps small teams move faster and make more progress than they ever thought possible.
Get Started - It's FREE* No credit card required
π οΈ Frequently Asked Questionsβ
Here are some frequently asked questions about "Predictive Analytics in Project Scheduling".